State of Search
What Is a Knowledge Graph, and Why Does It Matter?
Here’s the simple definition of a knowledge graph: It is a brain-like, structured database that stores facts — with flexible, bi-directional relationships. You’ve likely heard the term knowledge graph used in reference to Google or other search engines. This is because it’s the knowledge base that search engines use to furnish their results with information […]
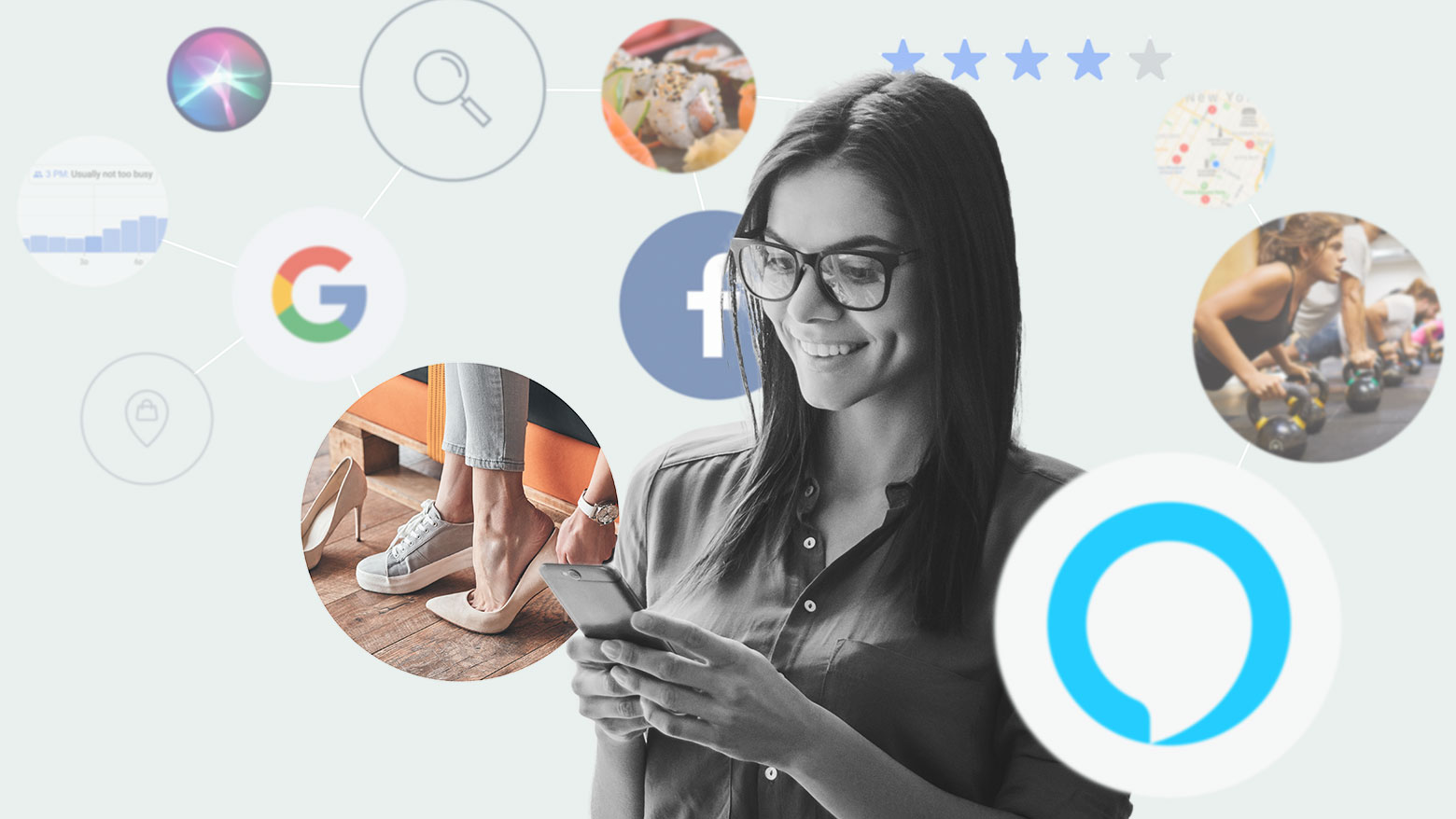
Here's the simple definition of a knowledge graph: It is a brain-like, structured database that stores facts — with flexible, bi-directional relationships.
You've likely heard the term knowledge graph used in reference to Google or other search engines. This is because it's the knowledge base that search engines use to furnish their results with information gathered from a variety of sources. Back in 2016, Google announced that its knowledge graph already held over 70 billion facts — and that's just the tip of the iceberg.
But knowledge graphs aren't only for search engines. While the term is most commonly associated with Google, it refers to any brain-like structure that stores facts (or entities) in this manner.
Why does a knowledge graph matter to your organization?
Advances in AI have made search engines "smarter" — allowing them to draw on their own ever-expanding knowledge graphs to answer users' questions with a higher degree of specificity than ever before. This has reframed consumer expectations, with people now being retrained to ask for exactly what they want. As a result, your organization needs to be able to answer these types of multi-dimensional queries with the specificity of a search engine.
Let's use an example: A potential patient performs a highly specific search for "best dermatologist near me open Saturday who takes Cigna." These types of questions can be difficult to answer, because the answer requires information from across your organization. This example query draws on ratings ("best"), specialty ("dermatologist"), office location ("near me), hours of operation ("Saturday"), and insurance accepted ("Cigna"). But answering it matters immensely since natural language phrases, while lower in search volume, are far easier to rank for and convert. In fact, natural language phrases have been shown to convert at 2.5x the rate of single keywords.
But you can't answer a question this complex if all of the specific details required aren't stored as related entities. To truly "speak the language" of search engines, your organization needs its own knowledge graph in which to store your own facts, all mapped to one another. This is the foundation for leveraging artificial intelligence in a martech stack and answering complex questions everywhere consumers search.
By doing so, search engine knowledge graphs can draw on the personalknowledge graph that you maintain — allowing you to manage your information at scale and deliver the highly specific answers customers now expect, both on your own site and across third-party experiences.
Learn how your business can build its own knowledge graph withYext.