Industry Insights
AI Is Your Copilot Assisting (Not Taking) Your Job
Setting up use cases for AI in your workflows can help you work better — and reduce risks.
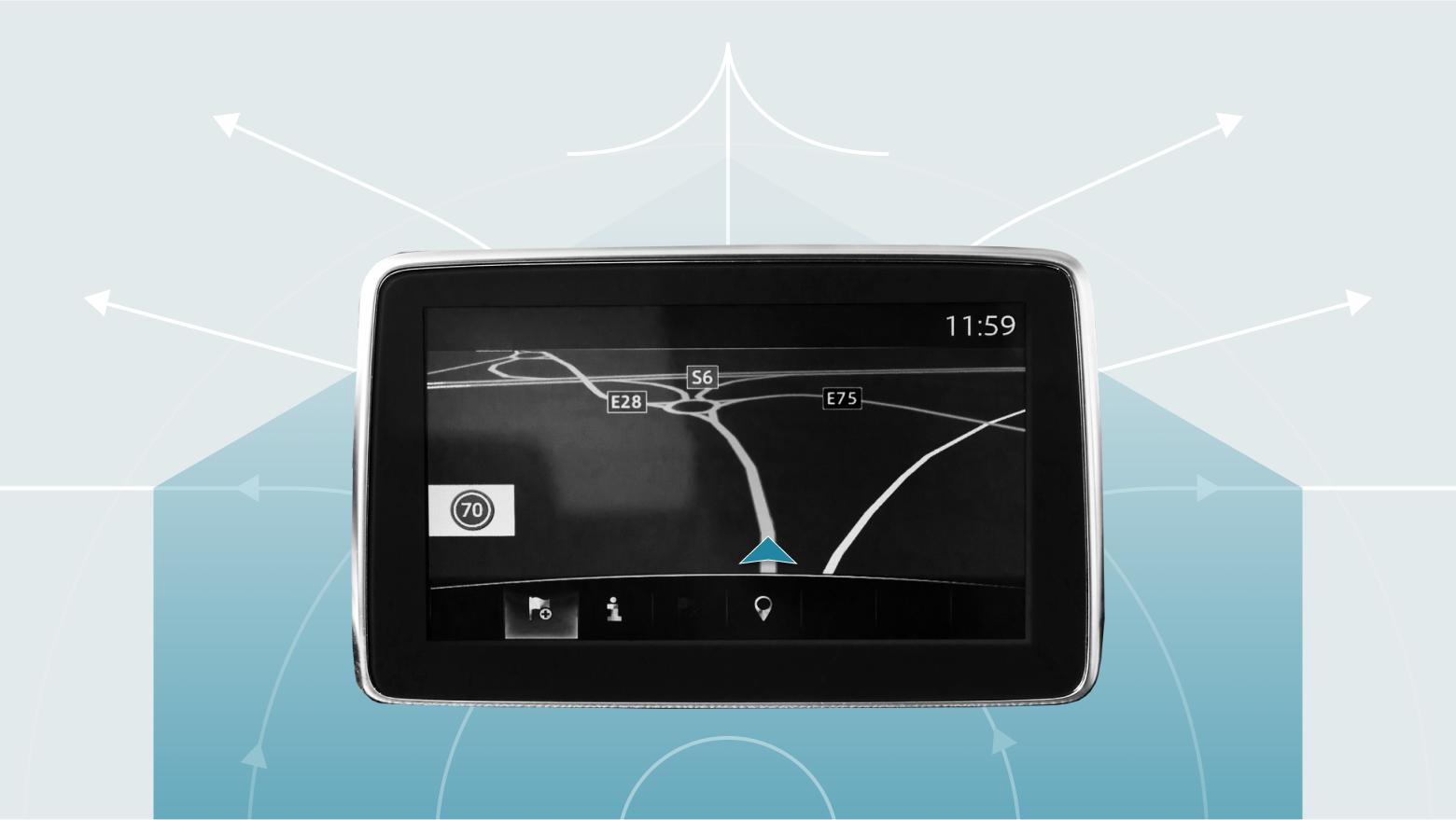
For all of 2023, large language models (LLMs) like GPT-4 have dominated news cycles. But throughout this period, the idea that a model might hallucinate — i.e., make up information when answering a user question – has been a primary concern. "How can we trust that the model won't make mistakes?" "What do we make of the fact that ChatGPT can get it wrong?'" These are valid questions. But the premise they're based on — namely, that the models powering experiences like ChatGPT are meant to be infallible — is what's wrong. Not the models themselves. That's because "hallucinations" aren't a bug: they're a feature. LLMs aren't static databases with all the answers. Rather, they are translation layers — interpreters — that can help us sift through information, reason better, and accomplish more than we could on our own. Sam Altman, CEO of OpenAI, said it himself on a recent podcast: "too much of the processing power, for lack of a better word, is going into using the model as a database instead of using the model as a reasoning engine." Okay, very well. But what's a business leader to do about this, anyway? And how can you leverage AI in your workflows while actually reducing risk? Leverage LLMs on top of structured data — don't crawl the whole internet The basic answer is that you can't treat LLMs themselves as a database that has the answer to every question. That's because data changes constantly. There may be some facts that stay true forever — i.e. The answer to "who was the first man on the moon?" – but there are huge swaths of information that are always in flux, from stock prices, to the weather, to the number of employees who work at a company. Thus, the best way to leverage AI effectively today is not to crawl the internet at large with an LLM — but to put together an underlying data structure that makes sense. LLMs can then draw on this unified data structure to deliver more accurate answers to users questions. Many businesses' data sits in a wide variety of places: it can be in different databases, or it can exist with outside vendors (like Salesforce or Adobe, for example). The key is finding a way to bring that data together — in a single source of truth— so that it can holistically power ALL AI experiences. Once your underlying data is ready, audit your workflows Next, devising highly specific use cases for AI in your workflows can help you reduce risks (without losing AI's benefits). In other words, forget the Star Trek of it all: AI isn't your captain. It's your copilot. Think about it this way: in the past, autopilot was considered a risk, but now it's vital for pilots. You should think about AI in similar terms. It's all about augmented — not artificial — intelligence. AI should be assisting your job, not taking it. Four concrete use cases for your AI copilot Once all of your business data is structured and stored in one central place that LLMs can "read" and pull from, you're ready for the next step: concrete use cases. To start, consider use cases for AI as your co-pilot within these four realms: marketing, support, commerce, and/or workplace. For example… In marketing, generative AI can be used as acreative assistant to write ad copy or meta descriptions. In support, AI chat can take the form of a chatbot for your support agent that helps them answer customer questions more quickly.. In commerce, AI can help your content creators write product descriptions, CTAs, and the like. In your workplace, AI chat can be used to answer employee questions — or automatically create a list of internal FAQs. The practical use cases of AI will continue to grow over time. But for now, savvy business leaders should focus on getting their business's information centralized and structured for interaction with LLMs. Then, they can set up basic workflows that leverage LLMs for their best use cases. Ready to learn more? Click here to read our full guide, Four Use Cases for AI.