Document Search with Extractive QA
Yext's Document Search algorithm searches through long-form unstructured documents (like blogs, bios, support articles, product manuals) and returns search results based on relevance to the query — it will even deliver a direct answer in the form of a featured snippet! Learn how Yext can help answer your customers' questions, the modern way.
Search Unstructured Data
In search, your goal is to get users the answers to their questions as seamlessly as possible. The problem is that your data exists in different structures. Some data is highly structured in your CMS, some in semi-structured FAQs, while other data is completely unstructured like a blog, help article, or web page. Document Search is an algorithm specifically optimized for searching long documents, to help you deliver answers to your customers.
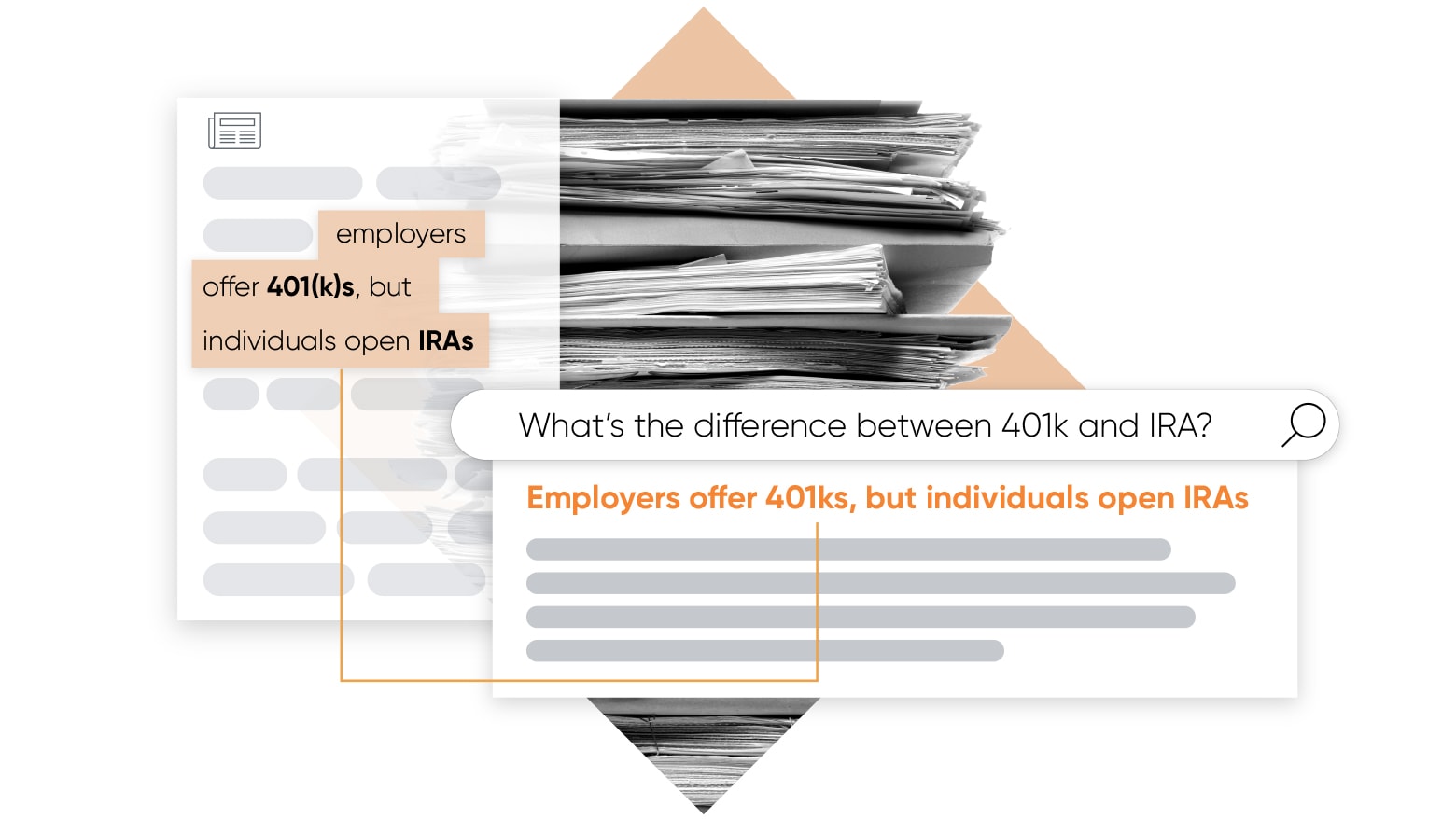
Surface Content Stored in Files
Document Search is able to index the unstructured content within a file (meaning the words in the PDF, powerpoint or document) and surface the relevant results and snippets in response to a query. This offers flexibility if you prefer to store an entire file in Yext Content, instead of extracting and storing the text as its own field on an entity.
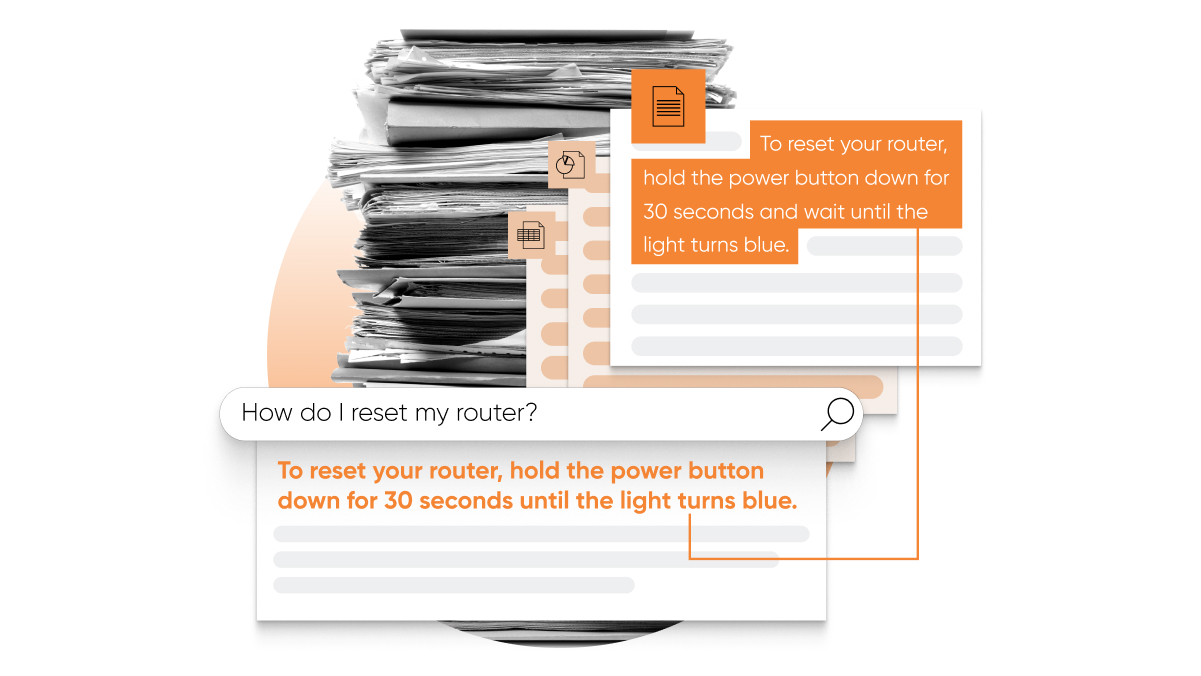
Provide Direct Answers Using Extractive QA
Extractive QA, like many other parts of the Search Algorithm, uses Google's open source neural network, BERT, which is trained to understand language. In Extractive QA, a specially trained version of BERT helps to identify the excerpts from long documents that best answer the user's question. If it finds a good answer in the text, the algorithm displays it as a featured snippet.
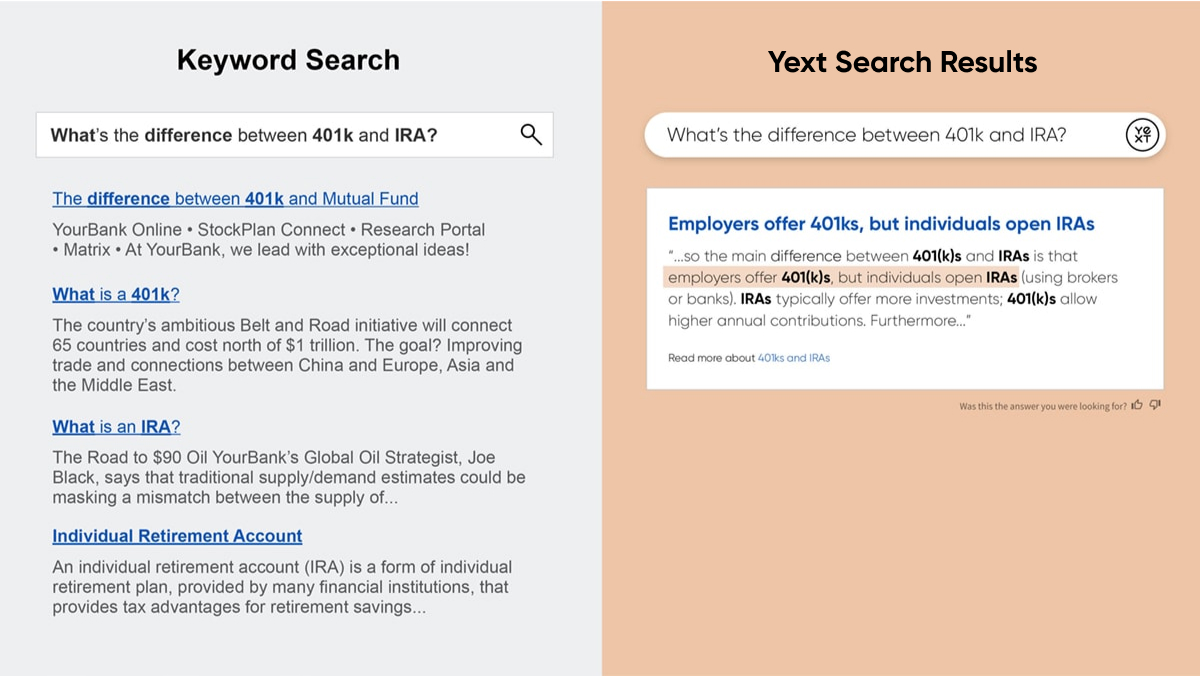
In-line Snippets
In addition to providing a direct answer at the top of the page, Extractive QA also highlights relevant context as in-line snippets on each result down the page. These snippets provide a great search experience and help searchers find the best answers to their queries, even faster.
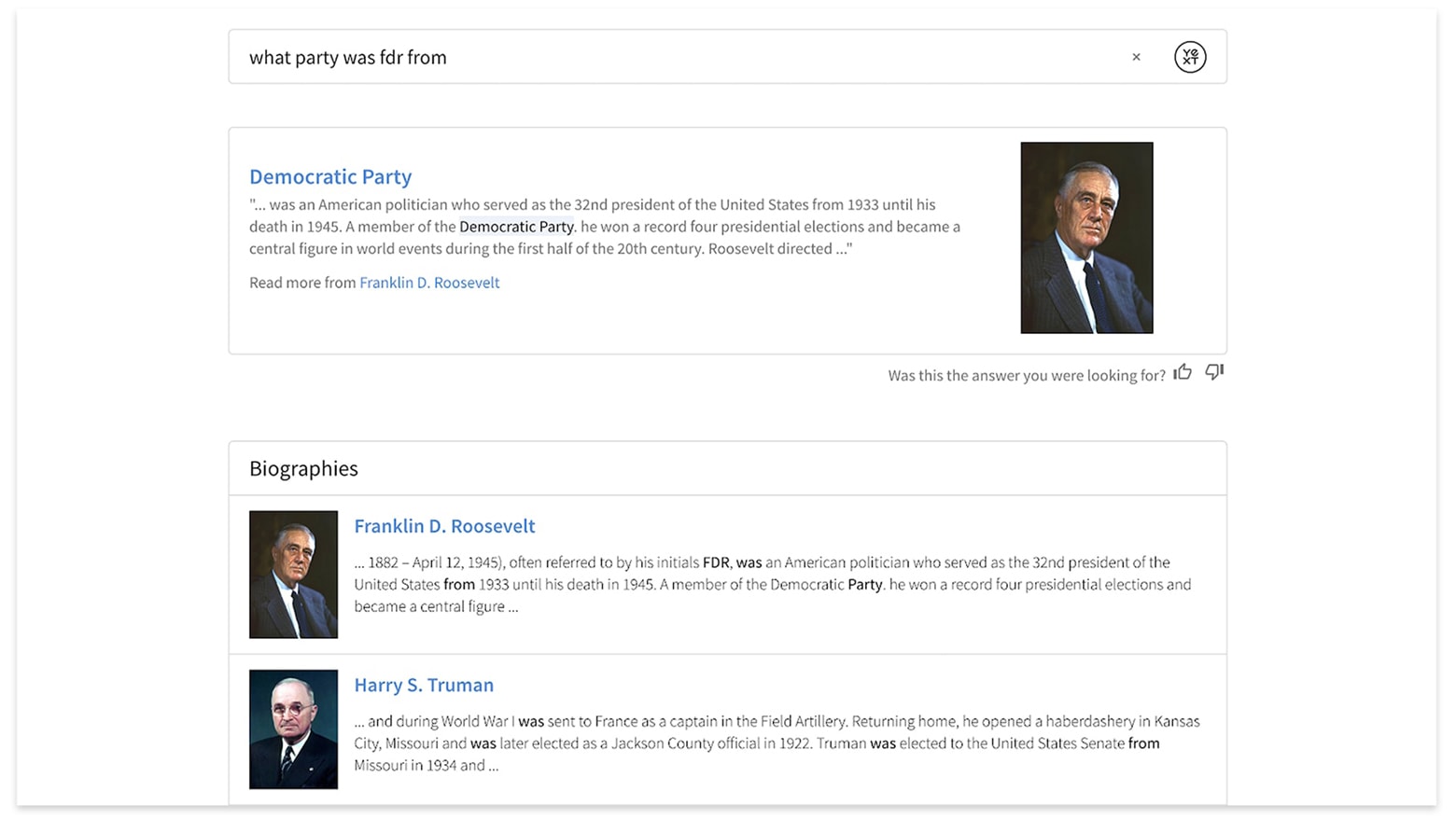
Capture Unstructured Data with Connectors
Unstructured data can be found across a number of different environments or sites. Places like your blog, your support site, or your press release hub may host a ton of great content that just isn't set up for modern search. Connectors like the Yext Crawler or Zendesk Connector can pull long-form unstructured data from apps or sources like your website so that you can build out your CMS and use it to start powering Search!
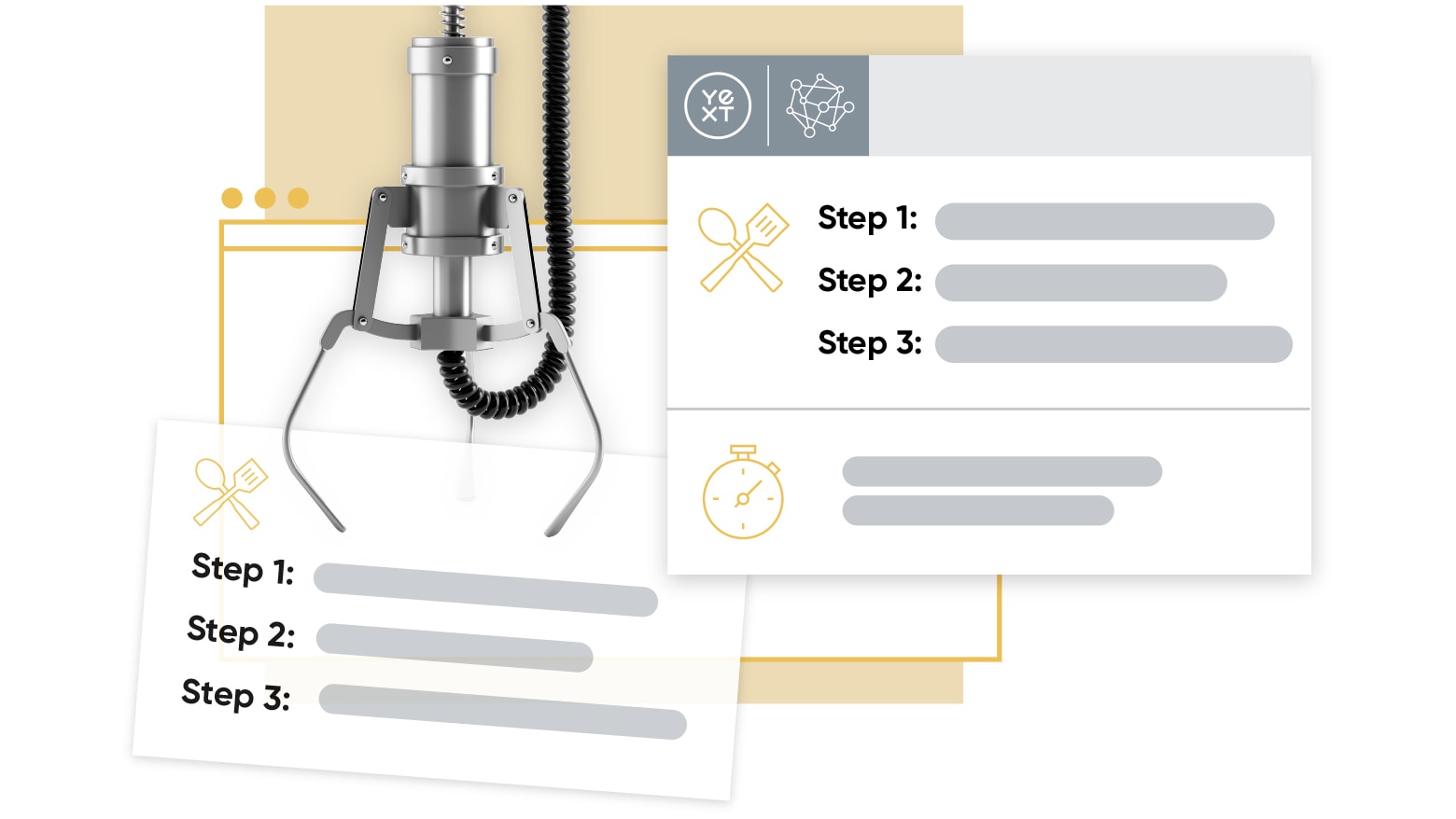
One Platform. Unlimited Solutions.
Drive revenue and delight customers
Transform site visitors into customers and learn more about what matters most to them with a dynamic AI site search on your website.
Capture and convert more local business with location listings. Turn maps, GPS apps, and local directories into your best marketing channel.
Your online reputation can win or lose you business. Generate, manage, and respond to reviews at scale with Reputation Management from Yext.
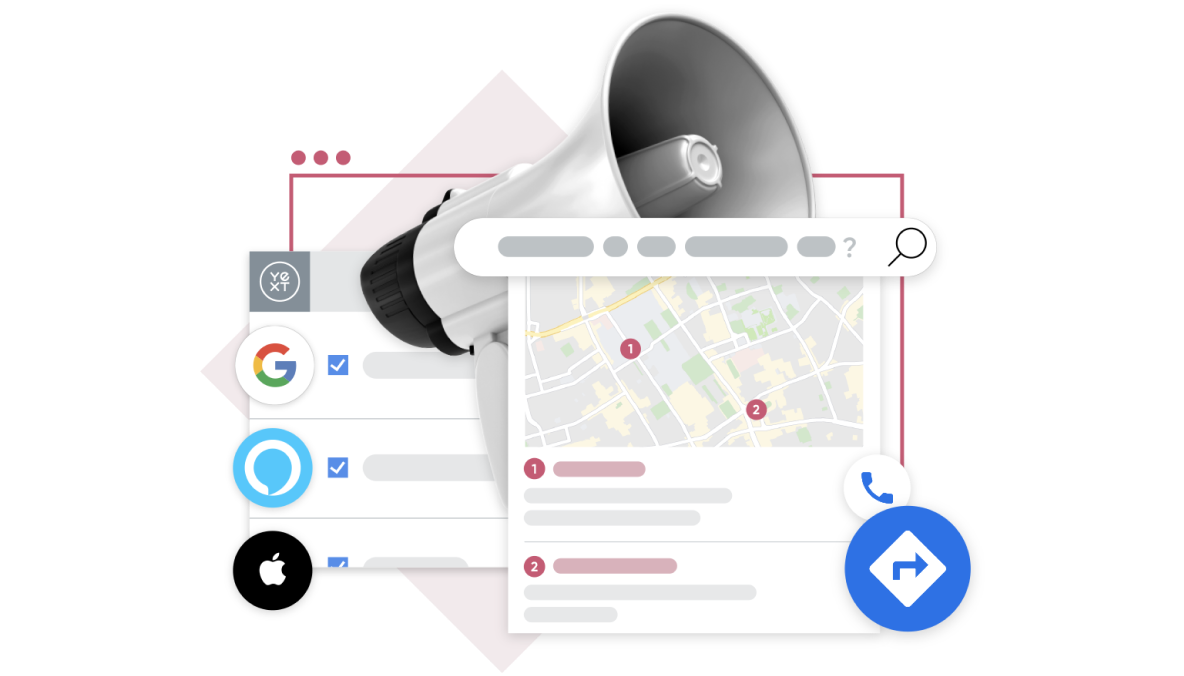
Empower customers and agents to self-serve
Enable customers to find instant answers to their support questions via FAQs, guides, tutorials, videos, ebooks, manuals, and more
Decrease case creation by recommending related content based on the issue being raised - right within the case submission form.
Resolve cases in record time with agent desktop search. Empower agents to drive faster case resolution as they troubleshoot customer issues at scale.
Answer your customers’ questions before they even reach your help site by improving your ranking and presence in search.
Deliver a seamless experience with in-app support. Allowing customers to seek help right within your app makes for a superior user experience.
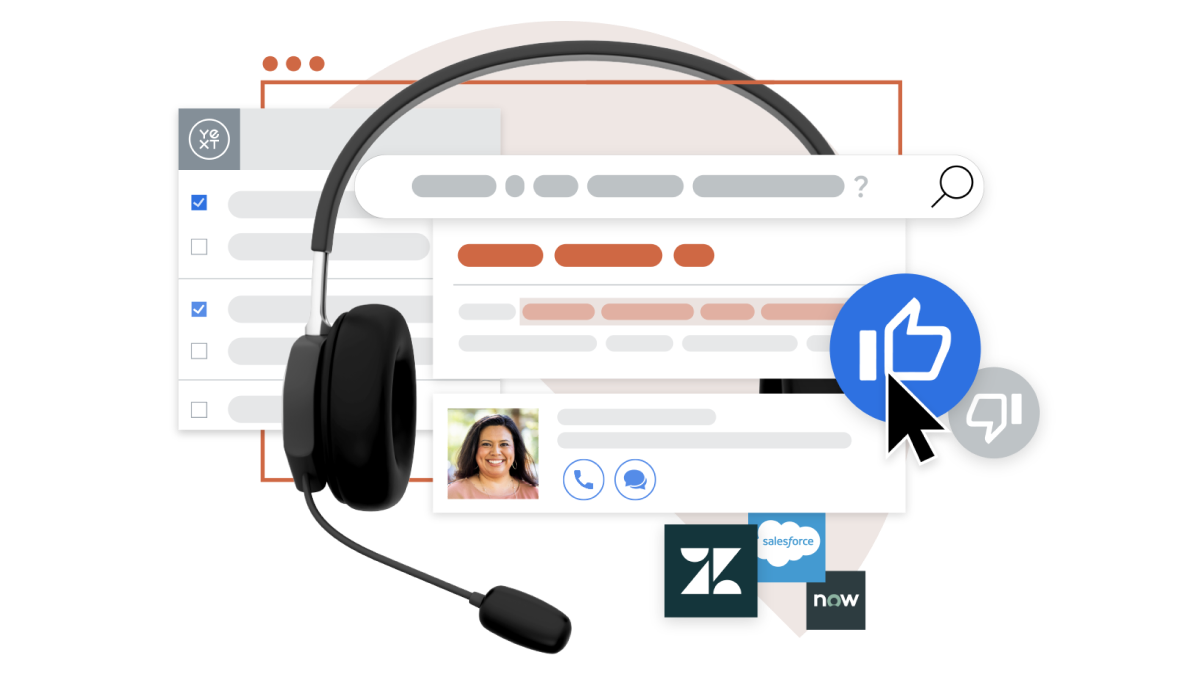
Increase sales
Connect customers with relevant products, services and experiences from search to checkout.
Yext Pages has powerful tools to let you confidently launch, manage, and scale your business, by building stunning detailed product pages that capture high-intent traffic.
Boost your local online presence with Yext's local landing pages. Easily create and manage customized pages for each of your business locations, complete with accurate information, photos, and calls to action. Attract more customers and drive more sales with Yext's local landing pages.
Create an in-person sales experience online that available 24/7. Guide your customers towards the right products or services that align with their individual needs.
Make it easier for customers to find your products by connecting your physical and digital storefront.
Tailor product data in bulk to easily update information wherever customers shop your inventory.
Effectively manage all of your product data in one place and easily share details to third-party marketplaces.
Build shopping excitement and trust that fosters more purchases.
Turn each interaction into a long-lasting relationship by connecting better with shoppers at every step of their customer journey
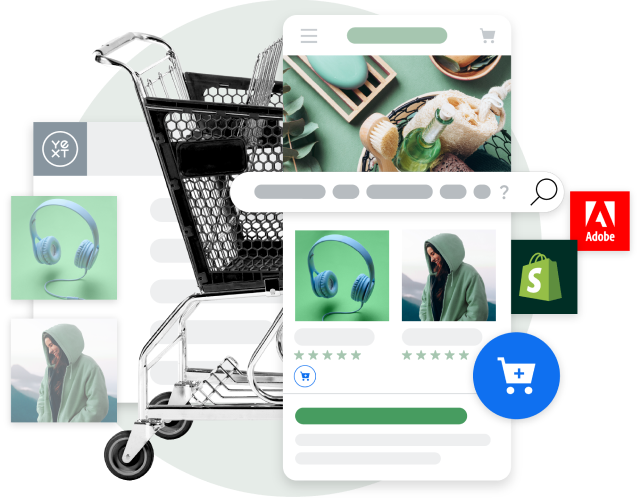
Improve employee productivity and satisfaction
Employees need company information to do their jobs. Make it easy, fast, and fun to find with AI-powered workplace search solutions from Yext.
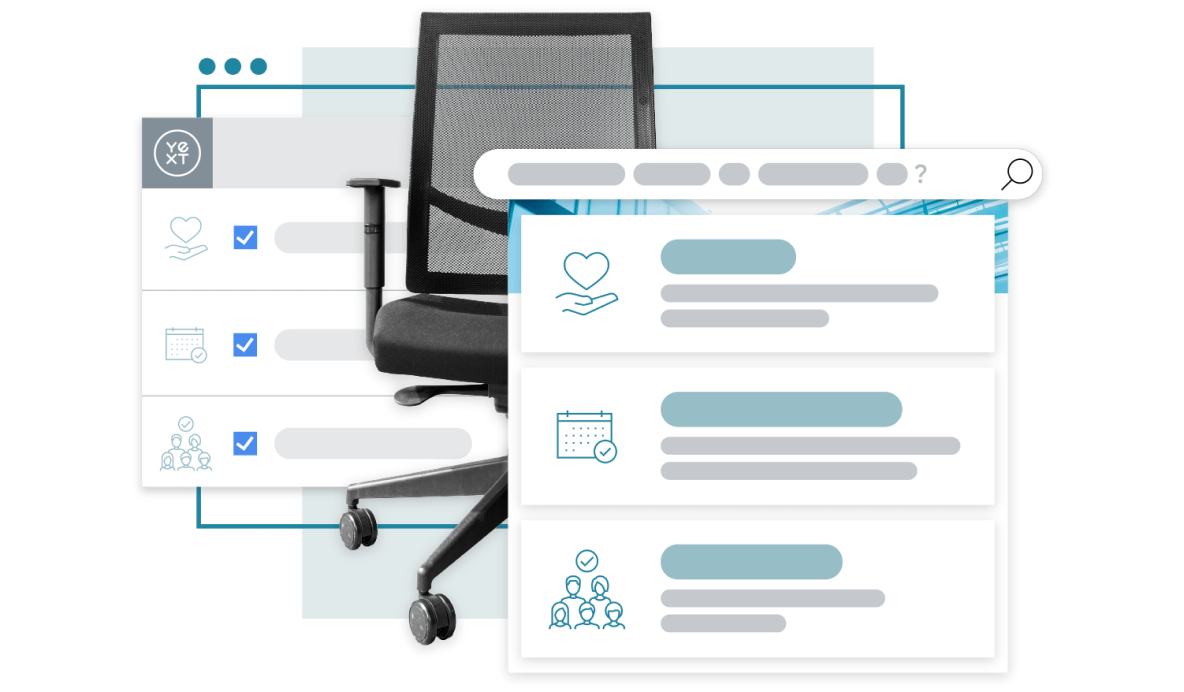
Build custom experiences fast
Build on the Yext platform for a fully custom AI search experience — fast. With SDKs, APIs, and robust documentation, the Yext Answers Platform provides the building blocks to create a bespoke search experience.
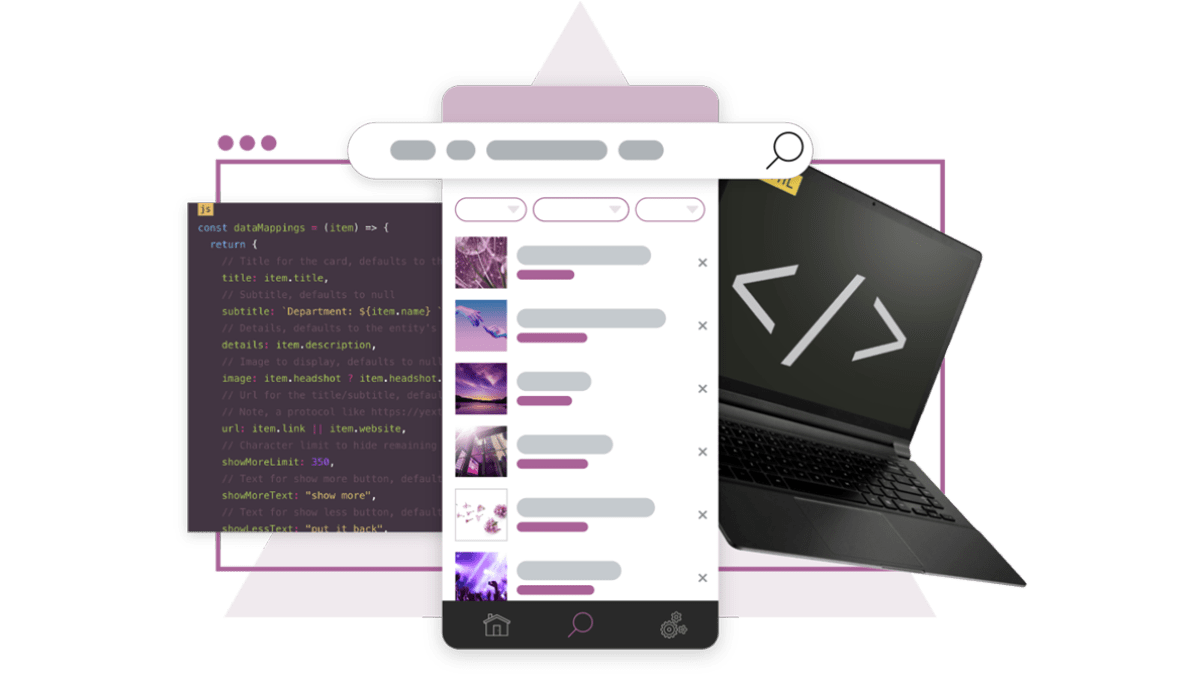
Explore Related Features