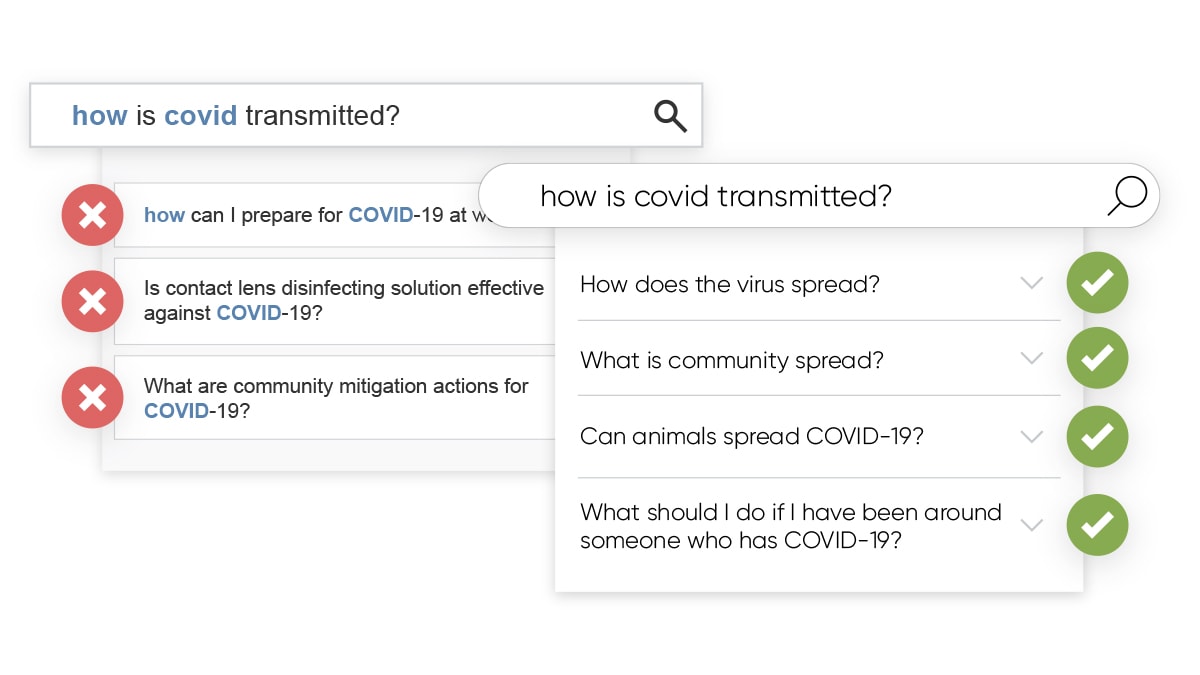
The Problem with Keyword Search
A great search engine understands a user's intent and returns the most relevant results. Modern search engines, like Google, Bing, and DuckDuckGo, are really good at this. But outside of major search engines, most enterprise search technologies fall short because they only look at the words in the query, not the intent behind it. They use "keyword search," technology that has not changed for decades.
But keyword search has a major flaw: humans use different words to ask the same questions. Consider the following examples:
The users behind these searches have similar or the same intent, and a keyword-based approach will fail to provide the most relevant results for these searches that are looking for the same information.
Keyword-based systems often employ techniques including TF-IDF, synonyms, stemming, and lemmatization to improve results, but these hacks are time-consuming and error-prone, and they still do not get to the intent of a user's search. The user will likely get a list of links that have at least one keyword match, and they will have to search for the relevant information themselves, if it is even there.
How Semantic Text Search works
We trained Google's BERT to better understand what a customer is really looking for. This means that, unlike keyword-based systems, Search knows that someone searching "send back shoes" is looking to kick off a return process, and that someone asking about a "dislocated shoulder" probably needs an orthopedist.
Search analyzes the meaning behind the query and finds the entities that have the most relevant answers to that query. It uses neural networks to understand precisely what the user is looking for and find the most relevant results from Content.
Yext fine-tuned BERT to better understand search queries. When a user enters a query, Search encodes it as a vector, creating a numerical representation of the query. Then, it looks for the data in Content that is closest to the query in vector space.
Two vectors that are close in space share meaning more than two that are far apart. Semantic Text Search places a query in vector space, and it locates the content that is closest to it, giving your customers the answers they might not have realized they needed.
These vectors have 768 dimensions—which is impossible for us to wrap our heads around—but you can visualize the process in two dimensions here:
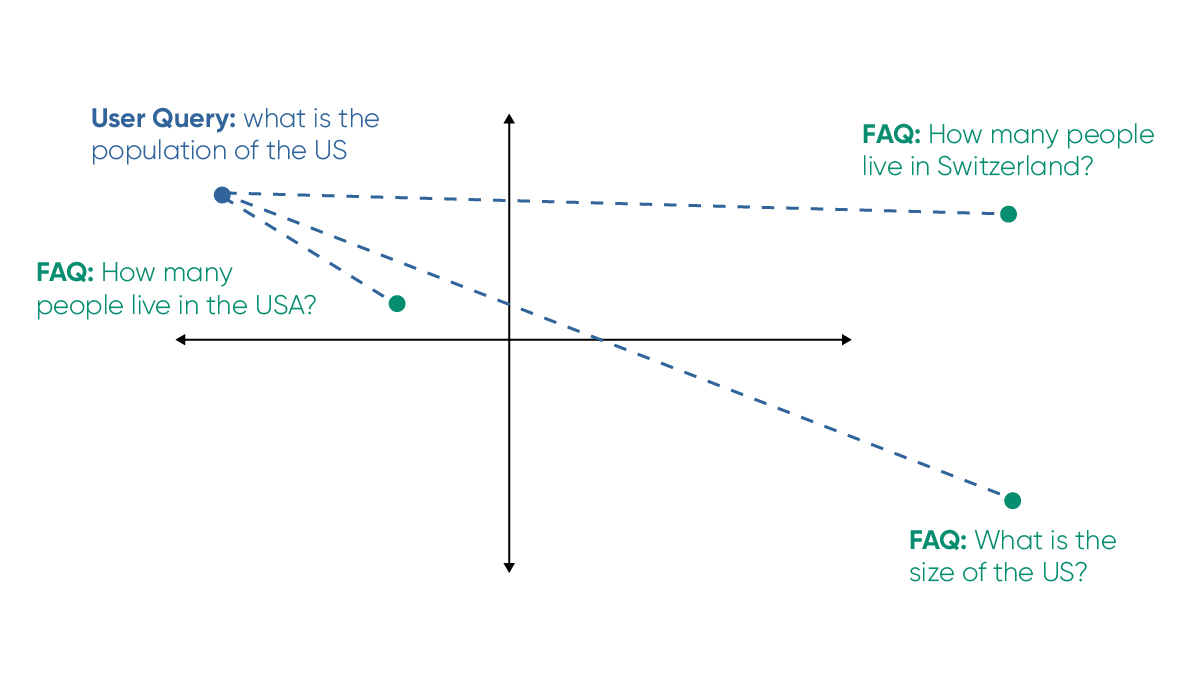
Explore Related Features
Explore Related Features